Statistical innovation could elevate global clinical trials: Cytel
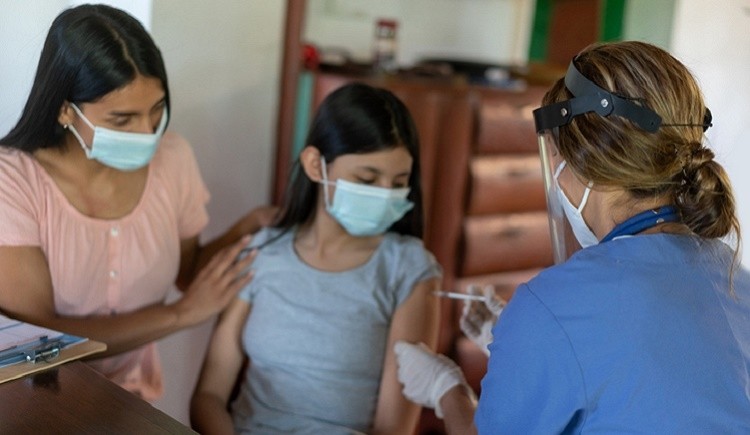
The populations of low- and middle-income countries face a disproportionate of the world’s disease, which further complicates efforts to catch up with the rest of the world in terms of economic development and other progress. According to experts from Cytel, innovations in statistical methods could help researchers overcome these and other obstacles in conducting studies in such regions.
Kyle Wathen, vice president of scientific strategy and innovation with Cytel, shared with Outsourcing-Pharma his views on recent advances in innovative clinical trial design and how this could help organizations bring more new therapies to market, faster and more cost-effectively.
OSP: How do you feel the clinical research industry has dealt with the challenges of the COVID-19 pandemic?
KW: It is truly remarkable how companies and regulatory agencies have worked together to get effective vaccine trials designed and completed in an unprecedented timescale. This pandemic is unlike anything most of us have ever experienced, and yet the industry has come together and brought several highly effective vaccines to market extremely quickly.
COVID-19 made companies embrace innovative technologies to remove the time and resource barriers facing them. Where organizations previously favored (or tolerated) traditional tried and tested techniques, regardless of their flaws, this immediate and urgent need forced organizations to explore novel designs and statistical methods.
For example, clinical trials incorporating Bayesian techniques have been deployed, delivering fast and effective results. These are just the first steps in exploring what innovative statistical techniques and clinical trial software have to offer, and we will see the long-term benefits way after COVID-19 is resigned to the history books.
OSP: As vaccine rollout progresses and the pandemic seems to be drawing to a close, what challenges still need to be dealt with?
KW: COVID-19 has touched every element of our lives, and whether patients have been infected with the virus or not, there is likely to be a lasting impact on patient health. We are already seeing patient drifts in populations as a result of patients being forced to delay treatment or health screening appointments.
This impacts trials in a fundamental way because when patients come into a trial with more progressed conditions, it can be hard to compare these results with earlier trial data. This could then impact how we make decisions in the clinical trial design process. As patients enter trials with more progressed diseases, we will need to adjust the design process so that it accounts for these potential patient drifts.
The target disease is not the only thing that will impact results; we already know that mental health issues have increased during the pandemic. Conditions such as anxiety or depression can alter patients’ quality of life and, combined with greater disease progression, are expected to have an impact on clinical trials. But, predicting the impact is very difficult. The pandemic certainly isn’t in the rearview mirror yet – we will feel its effects for years to come.
OSP: Why are the Cytel team and its partners calling for statistical innovation to mobilize improvements in clinical research?
KW: The traditional approach to clinical trial design is based on extremely old models and approaches that weren’t even created for medical research. In the race to get new drugs to market, organizations are under pressure to design trials at greater speed and deliver results quickly. This can cause teams to jump straight to the tried and tested models, making simplified assumptions (which may not be valid) and avoiding some of the complexity that comes with exploring innovative designs.
Of course, this complexity has been a massive barrier. In fast-paced clinical programs, there often isn’t time to take months or years exploring novel designs and employing the deep statistical expertise needed to execute these plans.
To meet deadlines, biostatisticians have thus taken a small selection of available designs and tested them in limited clinical scenarios. However, this approach disregards a whole landscape of designs that could be much more effective and help the trial reach its goals sooner and more efficiently.
Now, with the recent explosion in cloud-based computational power, trial design analysis that would have taken weeks or months can be completed in hours. This crucial development removes the barrier to exploring the huge complexity of potential trial designs that hold the promise of making studies more efficient and effective.
We know that when statistical innovation is used, more efficient clinical trial models are created that can generate superior results. By evaluating the design and operation of trials under realistic conditions, and simulating potential data that match expected trial data, statistical innovation can really add valuable insight beyond what has been done historically.
OSP: Which approaches will help achieve these improvements?
KW: Overall, we are gaining a better understanding of natural history and natural disease progression, and when clinical trials use these data in their design and operation, we see more accurate predictive simulations. By using relevant historical data, we can break original assumptions and create a precise picture of how a trial is likely to progress in these situations. Designing a trial in any other way is likely to result in incorrect decisions that lead to ineffective and inefficient trials. No one wants a superior treatment to fail in a clinical trial because of the trial design.
OSP: How do clinical research challenges vary from region to region?
KW: Similar clinical trial design challenges apply to every region, but some regions have additional challenges. No matter where the study takes place, it must be well thought out and focused on addressing the underlying purpose of the trial. Bridging studies have a role to play in regional trials and can provide an understanding of variations using statistical models and underlying biological data.
There are added challenges when conducting randomized clinical trials in low- and middle-income countries, though. Often trials are not properly planned or resourced to meet the needs of these regions, making it difficult to recruit eligible patients, gather robust data, and deliver improved outcomes.
We see statistical innovations, such as Bayesian methods and platform trials, as uniquely positioned to help with these issues. By simultaneously evaluating multiple therapies across geographical regions, with the historical data and understanding to provide context, trials can be made more efficient and cost-effective while delivering better patient safety.
OSP: What is an ’adaptive platform trial’ and will this approach improve operation and trial results?
KW: A platform trial is a type of design structure that enables the evaluation of multiple treatments and treatment combinations in a heterogeneous population. Such designs also allow new treatments to be added as the trial is executed.
Adaptive platform trials take that one step further by allowing predefined adaptions to be applied based on accruing patient data. When you design, set up, and start an adaptive platform trial, you plan for the trial to be able to evaluate treatments that don’t yet exist.
There are many benefits to this approach. Firstly, by evaluating multiple treatments in one trial, you improve operational efficiency. It’s much more efficient to set up a trial if you can test more than two different treatments; sites are more interested in participating if they know that there are wider opportunities with the potential for further therapies to be added.
However, the biggest benefit sits firmly with the patient. As the trial progresses, innovative statistical analysis and modeling enable the benefits of randomization and treatment comparison to be realized with smaller control groups. This means increasingly fewer patients are treated with the control as the trial progresses.
This is just one way in which trials are adapted – we’ve also seen some great work with patient sub-groups. For example, when patients are showing good responses to treatments, the algorithms increase the likelihood of new patients receiving effective treatments.
By using advanced statistical techniques, we will see trials flexing to accommodate the needs of the patients and their response to treatment. Of course, adaptations are pre-specified at the very beginning of the trial, so at no point does it veer off course; the trial simply adapts to the pre-defined qualifications determined in the design stages.
Designing a trial of this nature requires an incredible amount of expertise and experience in both frequentist and Bayesian techniques. But with the use of in-trial decision-support tools, the creation and evaluation of designs that lead to these complex, yet hugely efficient and effective, trials can be accelerated, enabling biostatisticians to add further value to the design process.
OSP: How will increased adoption of Bayesian techniques help elevate clinical trial operation and outcomes?
KW: Adaptive Bayesian techniques are the most natural way of including historical and in-trial data to inform and adapt the course of the trial and provide a more intuitive interpretation of the results. Adaptive trials use Bayesian techniques because they enable considered in-trial changes.
Clinical trial managers can quickly decide whether a treatment looks to be more beneficial than a control, allowing the flexibility to move resources and patients to more effective trials if needed. Direct comparisons can also be made against available treatments, and if the new drug is shown to be less effective, then trials can be stopped early.
In addition, Bayesian techniques allow you to make statements that are easily, and correctly, interpreted by many people – including those not highly trained in statistics. For example, a Bayesian approach allows you to make statements like, the probability the new treatment is more effective than controls is 98%. Using traditional methods, you could not make this type of statement.
OSP: Is there anything else you like to share about innovative clinical trial design?
KW: One of the most important things the industry needs to address is how we see the role of the biostatistician in clinical trials. Designing a clinical trial needs to be a team effort with the biostatistician integrated as a central part of the team, alongside clinical, operations, and patient advocacy groups.
Biostatisticians have been stifled with limited methods and computational power, meaning they can only explore a few design options and are often only consulted at the beginning and end of a trial. Eroding these barriers has been a key focus for us in the development of our enabling technology platforms.
With innovative statistical methods and the ease and power of cloud-based computing, biostatisticians can evaluate an expansive design space and explore innovative designs for more effective trials that deliver treatments more swiftly.
For example, our Solara system is a clinical strategy platform that not only provides this enormous computational power but also the collaborative workspace to allow cross-functional discussion during trial selection. With this framework, the biostatistician has a comprehensive view of each potential trial design under consideration, the futility and success factors of every design, and the impact of each on the trial operation. By using visualization tools, team consensus can be made on the most effective trial design.
As organizations embrace the idea of innovation groups (diverse teams of stakeholders from across the clinical trial design and operation space) and access the tools necessary for fast simulations, biostatisticians are freed from more manual statistical tasks and can step into the strategic space, adding more value to the design and operation of clinical trials.
To do this, organizations need to move away from the mentality of designing a study in the way it’s always been done. When we find better, quicker, and more efficient ways to design trials, we are more likely to identify treatments that will ultimately save lives.