More than ever expected of today’s data managers: IQVIA
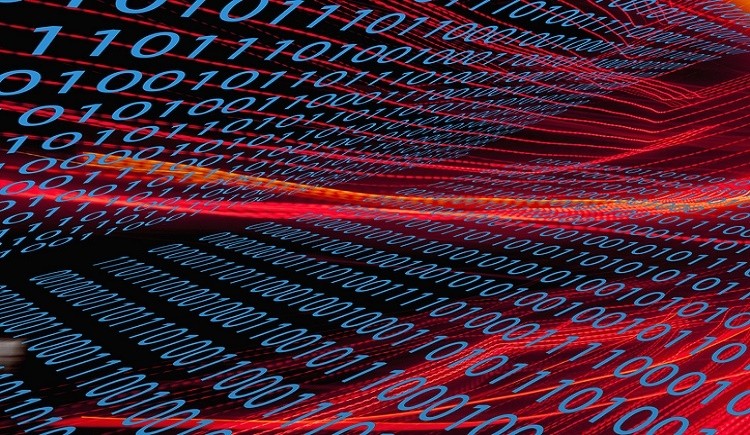
It’s not like clinical data management has ever been a job for the faint of heart. However, the role is more complex than it ever has been, thanks to advanced data collection and analytical technologies, regulatory shifts, market demands, and other factors.
Tracy Mayer—vice president and global head of biostatistics, clinical data management, statistical programming, and connected devices at IQVIA—discussed the ins and outs of a modern data manager’s role and technology that can help their work.
OSP: How have the roles and responsibilities of a data manager evolved over the years?
TM: As clinical trials themselves have changed over the years with more complexities and involving new technologies, processes, and regulatory demands, the role of clinical data manager has evolved accordingly. Clinical data used to be primarily collected in electronic data capture (EDC) systems, with 30% or less being derived from external data sources like labs and other vendors.
Today, on average, 70% or more of the data is collected from external data sources and only 30% or less is captured in EDC. Data derived from the external data sources often allows for more rapid access to higher quality data. But, this also means that data managers are now accountable for organizing and integrating all of the data collected from various sources like connected devices, electronic clinical outcome assessment platforms (eCOA), labs, and other vendors into one centralized dataset that can be used to analyze and report results. This requires a more technical approach to data management than in the past, as data managers deal with the specifications and requirements to allow for data acquisition to occur this way.
In addition, data managers must take a more holistic approach to managing the end-to-end data flow of the trial. These experts must have the ability to:
- Advise on a standards-based data collection strategy during study design
- Help manage compliance to the standards throughout the trial process
- Advise on complex study design from a database design and data collection standpoint
- Develop a data integration plan that allows for frequent access to data reporting and visualizations to inform more rapid and frequent decision-making.
Given the need for increasingly agile approaches, the data manager expert of today and tomorrow must be flexible enough to accommodate and advise on more frequent protocol amendments and postproduction changes. As decentralized trial (DCT) models, both fully virtual and hybrid designs, are used more often, these experts need to be comfortable supporting studies where there is greater reliance on technology solutions to drive interactions between site teams and patients. And, they need to have strong project management skills and experience to coordinate the multi-pronged effort required across a broader team.
As in the past, the role is still one that requires scientific expertise but has now evolved to require technical and informatics abilities as well as to ensure successful and quality delivery of clinical trial data.
OSP: Could you please talk a bit about clinical trial data strategy? What questions need to be asked at the starting line?
TM: All of the data-related trends like increasing complexity, more data sources, and the need to accelerate data delivery have been driving the development of innovative mechanisms by which we collect, aggregate, and analyze clinical information. The goal of every data management organization today should be to have a comprehensive data strategy in place that leverages robust standards, ensures operational compliance to them, and includes new technology to collect and aggregate clinical data beyond the EDC database. This helps with easier facilitation of processes driven by and reliant upon data flow such as real-time data cleaning, analysis and reporting, risk-based monitoring (RBM), and DCTs.
At the starting line, the study team and data manager have to think about what assessments and data points need to be collected, the types of patient interactions required, and at what time and in what order all will occur. They should also be thinking about what technology is best suited to meet study demands, including the data collection tools, like EDC and connected devices, that are required to acquire the endpoints. Also, regarding technology, consider what standards are available and/or need to be developed and what level/frequency of data ingestion and visibility to the data is required by the study team.
Finally, understanding the type of study design best suited to the overall goals of the entire program is key as well. It may be that an adaptive trial design will accelerate the program overall, but the study build must be done to facilitate that type of design from the start.
OSP: What elements should a solid data strategy include, and how might these components differ depending on the aspects of the trial itself?
TM: A comprehensive data flow strategy includes several key components:
- Data standards libraries for electronic case report forms (eCRFs), study data tabulation model (SDTM), and analysis data model (ADaM) datasets and a governance structure put in place to ensure compliance to those standards. Adherence to the standards varies dramatically between therapeutic teams and organizations, making governance critical to facilitate the proper use of standards.
- Tools and technologies to facilitate data collection from all of the data sources in clinical trials and to simplify the process of aggregating the data given various formats. These tools can also accelerate data ingestion through the use of application programming interfaces (APIs) that automatically pull the data into the data management system on a near transactional basis.
- Data strategists and other team members that are experts at integrating and aggregating data sources and overcoming the complexities of all of the various types of data coming from the tools. These experts can also work closely with vendors that supply data collection modalities (e.g., connected devices) to ensure the requirements and differences of each individual study are accommodated.
- A centralized repository containing the entire aggregated study data set that can be used to inform critical downstream processes in near real time like real-time data cleaning and RBM.
- Downstream processes that optimize the use of the dataflow strategy to drive accelerated trial delivery.
OSP: Please tell us a bit about how the amount of data available and complexity of the information have evolved in recent years.
TM: We are seeing a much higher rate/volume of data generation in clinical trials and anticipate that will continue to accelerate. Alongside the increasing complexity of study designs and the types of data sources being used, the complexity of the data (e.g. higher fidelity imaging data) has also increased.
We have also seen rapidly evolving dynamics with respect to the EDC systems we use in clinical data management. As mentioned earlier, we have seen a shift from 70% or more of study source data collected in EDC systems to 30% or less of the data being captured in these systems. The emphasis on other external data sources like sensors, connected devices, and electronic health records (EHRs) has taken over the majority share. And, automated, digital end-to-end processes are used more often to decrease the time and cost it takes to handle and validate the increasing volume of data in addition to increasing the quality of the final datasets.
OSP: Considering all the ways data management has become more complex, how can data managers and other folks work to ensure the data collected is clean, qualified, compliant, etc.?
TM: Standards and technology are two of the biggest advantages data managers can leverage to respond to the increasing complexity of trial data. Having a robust library of therapeutic data standards for database build and acquisition (eCRFs, edits, DMP) as well as standards for the analysis and reporting of the trial data (SDTM, ADaM, TLFs) allows for data managers and the study team to ensure they are capturing the data most relevant to the study endpoint(s).
More automated data management tools, such as digital workflows, ensure all of the data captured is being validated against the requirements of the data management plan. And, data collection technologies (e.g., EHRs, electronic patient-reported outcome (ePRO), sensors) ensure higher integrity data is collected directly from the source with less additional intervention required to validate the data collected.
Compliance with standards as well as the use of data collection tools that meet regulatory requirements is also critical. Having a data management team that has experience validating data capture tools and systems and expertise to know what systems/devices are approved for use in various countries is also important to ensure the data is compliant and qualified for use.
OSP: What can DMs do to speed the collection and processing of such data?
TM: There are many opportunities for data managers to accelerate the collection and processing of study data. As mentioned previously, therapeutic data standards allow the data managers to accelerate the database build process as predefined eCRFs and edits are already available to the team for use.
Data standards also allow for more rapid cleaning of the data with standard edit checks and data validation rules that can reduce database lock to less than two weeks after the date of last patient, last visit. Utilizing a device-driven data acquisition strategy is also advantageous. It allows for higher integrity data capture directly from the patient via tools like ePRO and connected devices that are available near transactionally to the study team. The automation of data transfers from external data sources, like lab data via the use of APIs, can allow the team to get daily uploads of new data, allowing for reconciliation and cleaning to occur immediately versus doing the activity once a month or quarter.
OSP: What do they need to consider, and what tools can they use, when gathering data from a whole range of sources (EDCs, wearables, etc.)?
TM: The most important consideration when selecting EDCs, wearables, or any other data vendor is the expertise of the vendor and the robustness of their solution. It is critical to select EDC vendors that have a solution that supports the trial design. For example, if an adaptive trial design is being used, working with an EDC vendor that can support randomization and trial supply management, and ideally offer this as an integrated part of their platform solution, is recommended.
When selecting a vendor to support connected devices and wearables, it is important to choose one that:
- Can advise on the devices best suited to collect the required data points
- Is familiar with regulatory requirements for devices in all countries in the study
- Can rapidly qualify and validate each device being used and can provide logistics and procurement services to ship the devices to the sites
- Provides ongoing maintenance and support
In addition, not every connected device vendor can offer the means to ingest and integrate data from more than one device on the same study. Sponsors may want to consider a connected devices provider than can do all of these things, while also providing data management support as part of a holistic connected devices strategy.
OSP: How can professionals from organizations like IQVIA help with all of these demands placed upon data management?
TM: At IQVIA, it is important to offer a holistic dataflow strategy that includes a robust data standards library with a governance structure and supportive technology to drive compliance to these standards to accelerate database build and lock activities. We also have a data integration and ingestion strategy that accommodates the rapid acquisition of data from any type of data source into a data repository where it can be aggregated and accessed on a near transactional basis to support our real time data cleaning processes and analysis and reporting activities.
Another key element is to provide a complete and dedicated connected devices solution to support study design, device qualification/validation, logistics and procurement, data ingestion/integration, and data management services specific to sensors and wearables.
Lastly, housing a deep level of expertise on our data management team, who can provide more support to the data manager and study team in dealing with the increasing complexity of clinical trial data, is also part of the plan. These roles include technical designers, who guide database development and optimize the use of standards, and data strategists, who develop a comprehensive data integration strategy for each study to enhance the ingestion and aggregation of various data sources.