AI can be a powerful tool in drug development, discovery: Tellius
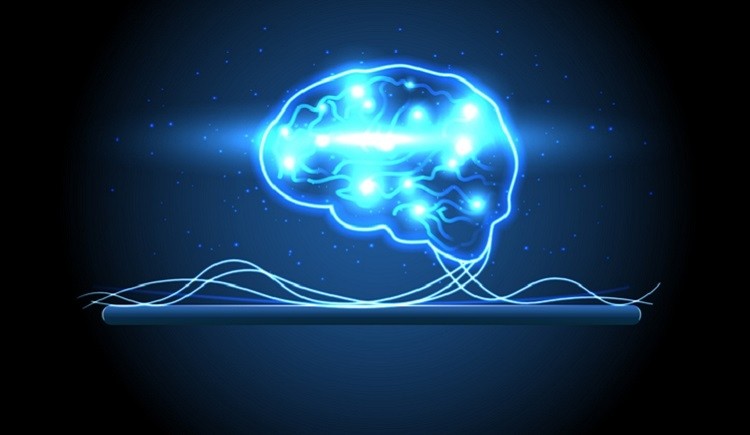
Professionals knee-deep in drug discovery and development usually have a wealth of data at their fingertips. The question of how to make the most effective and efficient use of the available data is another matter entirely.
Outsourcing-Pharma recently connected with Ajay Khanna, CEO and founder of Tellius, to discuss how advanced analytical tools like artificial intelligence (AI) can be put to work, to help make the best use possible of data.
OSP: Could you please share the ‘elevator presentation’ description of Tellius?
AK: Tellius is an AI-powered analytics and decision intelligence platform that enables anyone, regardless of analytical skills, to quickly ask and answer ‘what’, ‘why’, and ‘how’-type questions of their granular enterprise data in order to make better, faster decisions.
Tellius accelerates the journey to insights-driven decision making by providing a dual analytics engine that combines a natural language query interface built on a columnar search engine with machine learning capabilities. The platform allows for AI- and ML-driven automation that augments human analysis by analyzing billions of records and identifying automated insights such as trend drivers, root cause analysis, and anomaly detection.
Our platform is the only one capable of allowing you to essentially search your data for insights—asking questions such as, “Why were sales down in Q4?” and getting automated visualized answers to help you make valuable business decisions.
Tellius was founded in 2016, has raised $17m+ USD in venture capital, and has three of the top 10 global life science firms as customers, as well as several large CPG firms, multinational banks, and eCommerce and startups seeking to augment analytical capabilities and go beyond static dashboards.
OSP: Please share an overview of how the life sciences (particularly clinical research professionals and other drug development folks) have had increasing amounts of data at their disposal as collection methods evolve, and some of the benefits and challenges.
AK: In today’s increasingly digital world, pharmaceutical and life sciences companies have access to greater amounts and varieties of data than ever before. From hospitals, tech companies, and academia environments to EHRs, medical imaging, insurance records, wearables, and health apps, to social media, genomics, third-party anonymous patient-level data (APLD), and health data exchanges, data is abundant.
Life science firms that successfully derive insights from data to make better decisions outpace their peers via faster, more cost-efficient drug discovery/development and personalized medicine for better patient outcomes. Unfortunately, doing so is not easy with current tools.
There are numerous challenges in managing this continuous stream of data at every step of the data value chain:
- Storage and access: how do we securely/compliantly store & share all this data?
- Cleaning: how do we reconcile/harmonize/join these disparate data sources?
- Exploration: how good is this data? what is it telling us?
- Insights: why is this trend happening? what is driving it? which segments exist?
- Predictions: which outcomes are likely to occur?
- Sharing: how to share/translate this data story to business-minded colleagues?
- Monitoring: how to spot anomalies and monitor for changes?
- Action: how to make this data actionable (an app? embedded? PowerPoint?)
OSP: Could you please talk about how AI-centered analytics can boost clinical trial success, optimize the supply chain, and improve a pharma firm’s marketing efforts?
AK: AI-powered analytics and decision intelligence add value in numerous applications. For example, these technologies can boost clinical trial success by identifying suitable patients as well as monitoring trial enrollments and dropouts to speed up trials. Additionally, AI analytics and decision intelligence can increase patient safety by automating anomaly detection.
Across the supply chain, AI analytics and decision intelligence can identify the components impacting high cycle times to improve capacity planning, automate root cause analysis to reduce variation, and predict ordering patterns to optimize supply utilization. AutoML and predictive machine learning can also help prevent workflow delays that impact quality processes.
When elevating marketing efforts and boosting market share, AI analytics and decision intelligence are critical to performance tracking, sales force effectiveness, and market share analysis. For performance tracking, organizations can automate the analysis behind a drug’s performance in certain markets or HCP coverage areas. Coupled this tracking with ML algorithms fueled by prescription data and automated market share analysis can help companies make better sales and marketing decisions
Within pharmaceutical organizations, these innovations can support rebate operations and improve market access. From a rebate perspective, AI analytics and decision intelligence can identify cost-saving opportunities and shift rebate plans based on utilization, variance, and provider/patient reviews. Pharmaceutical companies can also improve cash flow forecasting from real-time insights into things like resubmission predictions.
When optimizing market access, AI analytics and decision intelligence can predict market impact based on changes in access and payers, provide real-time insight into brand performance. These metrics are also critical in identifying potential growth segments in new patient populations and regions.
Here's a sales force effectiveness case study: A young pharmaceutical company recently gained FDA approval for a rare cancer treatment. The treatment was only eligible to patients once they had undergone three to four rounds of other treatment.
Spotting eligible patients – or rather, spotting healthcare professionals (HCPs) who would consider prescribing patients this new drug – was a needle-in-a-haystack proposition. The pharmaceutical company’s limited salesforce couldn’t afford to indiscriminately cold call HCPs. They needed a targeted approach.
This company used augmented analytics to merge internal CRM data with third-party patient claims data to find similar classes of oncology drugs. They used that data to build and train a machine learning-based HCP targeting model to predict which accounts were most likely to become prescribers. This in turn provided them the focus they needed to conduct their sales outreach.
The outcome: significantly, the work was completed by business analysts on their existing data teams – not by advanced data scientists – and helped the firm identify 30% additional new opportunities, all without adding sales staff.
OSP: Please tell us about where you think the use of AI in clinical trials and drug development/marketing might be headed—what potential of this advanced tech has yet to be realized?
AK: Decision intelligence has enormous unrealized potential in the life sciences industry as AI has only been applied in the last few years. We expect even broader adoption of AI-powered analytics in each aspect of the drug development lifecycle in the coming years, including:
- Discovery: virtual “dry” labs via computer modeling for molecular discovery are growing and we expect analytics to grow around the field to optimize drug discovery.
- Clinical Trials: clinical trial data is notoriously tricky due to regulatory sensitivities. Despite this, we expect the traditional sequential clinical trial process will evolve to include AI-enabled analytics, reducing the ‘lab to life’ cycle via predictive insights to boost trial safety, optimize trial design, automate the delivery of data intelligence to trial workflows, and fast-track drug development.
- Commercialization: marketing and sales use cases abound for augmented analytics because ROI is more immediately measurable. In the future, we expect an even greater number of pharmaceutical firms to leverage this technology and tackle other applications such as market mix modeling (i.e., promotion response modeling) and even greater HCP outreach personalization.
OSP: What does your company have planned for the future—any new products, enhancements, interesting partnerships with other companies in the works?
AK: Tellius continues to invest in data connectivity capabilities (as new sources, exchanges, and third-party data providers emerge each year); augmented data transformation capabilities (since these data sources must be harmonized); greater ease and flexibility capabilities to continue to democratize data accessibility for all user types via no/low code features; deeper automated insights capabilities (for unmatched insight actionability); automated data storytelling functionality (to expedite communication to result in faster decisions); additional AutoML/point-and-click predictive analytics capabilities (to put the power of advanced analytics into the hands of more users); and partnerships with leading cloud providers such as Snowflake, Databricks, Looker, and big three cloud providers (Azure, AWS, Google) as well as leading global system integrators and consultancies.
OSP: Anything to add?
AK: Decision intelligence adoption is gaining momentum in the life sciences industry. Firms seeking to explore how to bridge the gap between dashboards, advanced analytics, and AI and machine learning should consider decision intelligence and keep in mind:
- It's okay to start small: Instead of a multi-million-dollar investment out of the gate, it's possible to pilot a few use cases or roll it out to a single division or a specific user type, then expand as value is proven and needs increase.
- No rip and replace: Decision intelligence tools complement traditional BI and data science tools, rather than outright replace them.
- More perspectives lead to better decisions: The big promise of augmenting a firm's analytics capabilities is the greater inclusion of perspectives and ideas unlocked by the democratization of analytics practitioners and the upskilling that this technology enables through intelligent automation. Better decisions lead to better outcomes and competitive advantage.
The future is certainly bright.