Predictive analytics could unlock precision medicine progress: AiCure
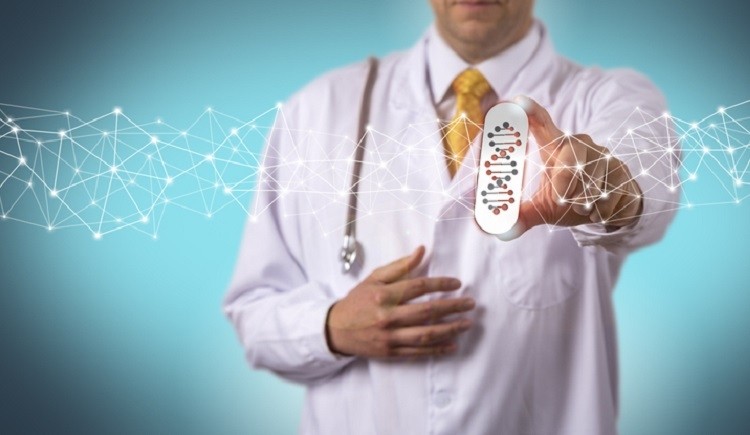
Harnessing predictive analytics can offer clinical trial teams a wealth of information. For example, using this technology could help inform sites and sponsors how patients might adhere to a medication regimen, respond to a drug, and more; these insights can help not only design more successful trials, but also to predict a patient’s response to treatment, craft personalized medicines, and more.
To find out more on how predictive analytics can be put to work in clinical research, Outsourcing-Pharma reached out to Rich Christie, chief medical officer with AiCure.
OSP: Could you please share how predictive analytics has been put to work in various aspects of research and care in recent years?
RC: Predictive analytics can play a significant role in understanding and anticipating participant engagement during a trial. By leveraging computer vision and AI to remotely assess patient dosing behavior and capture digital biomarkers, analytics can be applied to the audio and visual data collected. The resulting insights can be used to reduce trial risk and better understand and target medications to the right patients.
In a research study AiCure conducted, we determined that medication adherence can be used as a predictive indicator for both ongoing adherence during a trial and the likelihood of dropout. These insights allow sites and sponsors to implement strategies to reduce trial risk.
In some situations, two-week lead-in periods could be used as a way to stratify participants to ensure a balance across treatment arms, while in other situations determining the probability of dropout can reduce the need for significant over-enrollment. Additionally, using an individual’s predicted adherence can be used to customize support and intervention strategies so that resources can be properly allocated across trial participants to maintain adherence and reduce dropouts.
Capturing digital biomarkers during a trial also enables remote detection of subtle changes in a patient’s health status and response to treatment. Applying predictive analysis on top of all the audio and visual data collected during a clinical trial opens the door to having a much more precise understanding of a patient’s true experience with any given medication: from how they will respond to their medicine, to the likelihood of developing side effects, to the broader progression of their disease over time.
As predictive technologies continue to advance, the benefits will go far beyond anticipating how a clinical trial will progress to allowing clinicians to provide proactive, personalized care to patients at a scale that hasn’t been seen before.
OSP: Specifically, how can the use of such solutions help bridge the lessons learned in clinical research and apply them to real-world care?
RC: While clinical trials take place in a controlled environment, there is much to learn from the types of data we can collect during a study, as well as the insights they can deliver.
A drug’s market success is increasingly defined by patients’ lived experiences with their treatment. In patient care, dosing is often a proxy for a patient’s engagement in their treatment plan. By utilizing the same predictive analytics we employ in the research phase, we can highly personalize a patient’s real-world care plan.
Firstly, by understanding if patients are taking their prescribed medications when they are supposed to, clinicians can deploy patient support programs that are tailored to patients’ needs. If a patient has a complex drug regimen that is hard for them to follow, providers can determine if push notifications when it’s time to take a pill would help them or if the person would be better equipped if they were assigned a care manager to offer greater support.
Secondly, by implementing digital biomarkers in their care management, providers would be able to better understand the nuances of someone’s disease trajectory and whether a medication is having the intended effect. Monitoring the subtle changes in a patient’s condition would allow clinicians to make decisions about changing a patient’s dosage or prescription in a more timely manner.
OSP: Then, how can predictive analytics be of particular use in precision medicine?
RC: Predictive analytics is the key to truly making precision medicine a reality in healthcare. By understanding how a drug is working for an individual patient, clinicians can personalize care plans to best suit that patient’s particular needs and provide a new level of proactive and preventive care. Since many factors impact the effectiveness of a treatment, such as when a patient takes their medication or how their body responds, using predictive analytics can allow clinicians to monitor when a patient doses, assess their response and help a patient identify the right dose and more precise dosing timing to maximize the impact of their treatment.
OSP: How can predictive analytics be coupled with patient voice to help gain a better understanding of a patient’s journey?
RC: Many disease symptoms are visual or audible in nature. Using computer vision and AI to gather and analyze visual and auditory cues allows us to pinpoint critical patient responses and behavioral trends. For example, if a patient with depression has slowed speech, that may begin to provide a deeper understanding of their status and potential underlying conditions. A clinician may be able to identify that the patient is having a depressive episode and adjust their medication to improve their outcomes.
OSP: Please talk about how predictive analytics can help guide understanding and practices around medication dosing, prescribing, and other related aspects.
RC: We know from AiCure’s own predictive adherence algorithm that we can utilize rich information beyond if a dose was taken, including how long it takes a patient to dose, variations in the time of day they dose, how long after their alarm time they dose, and more. Achieving this level of insight in a clinical trial can inform future prescribing and timing for exactly when patients should take their medication based on their anticipated response.
For example, when and how a patient with Parkinson’s disease doses their medication – what time they take their dose, what they eat, their activity level, etc. – can significantly affect the impact of the medication. Predictive models can help clinicians and patients know the ideal dosing times for that individual patient to give them the best response.
Predictive analytics could someday also help forecast the course of a disease. For example, multiple sclerosis and Crohn’s disease frequently have a waxing and waning presentation over long time periods. With effective, long-term longitudinal data collection of symptoms across time, clinicians may someday anticipate flare-ups and disease progression to inform the future of a clinical trial and a patient’s care.
OSP: You mention that it can be used to anticipate treatment response and disease progression in a trial setting—why is that important?
RC: With a stockpile of high-quality data, predictive insights have the power to extend their reach well beyond adherence. By leveraging patient-level video and audio biomarker data, researchers can make significant strides in predicting how a patient will react to medication and course-correct in a timely manner if a patient isn’t responding as they anticipated.
A strong example of this is depression treatment. When creating a selective serotonin reuptake inhibitor (SSRI) therapy, many times participants must wait four to six weeks before they are reevaluated to understand the impact of that specific medication. By accumulating and analyzing the right data in a clinical trial, we could develop a predictive model that determines what biomarkers might make clinicians aware in two weeks whether a patient is likely to respond to a particular treatment and intervene much sooner.
OSP: Also, you bring up the concept of a “toolkit” of predictive knowledge—please share and tell us how that might be of particular use to both researchers and caregivers.
RC: Developing a toolkit of predictive knowledge during a clinical trial can give clinicians a general idea about whether a drug is the right fit for a particular patient. This is especially important for complex conditions with a wide display of symptom expressions.
With PTSD, for example, the disease has a very heterogeneous population, and there is no one-size-fits-all treatment. Predictive models in clinical research can help clinicians segment the population based on which medications they may respond best to, and which dosing patterns help enhance their response. As those same individuals go into the real world and use the same smartphone-based tools, researchers can continue to check back on those learnings and develop inferences about their wellbeing, notifying clinicians when it may be time to back check in with them.
OSP: Adherence to a clinical treatment regimen can be challenging—can you share how trial teams can determine patient-specific support strategies and put those in place to improve adherence and reduce dropouts?
RC: When we have access to a patient’s past behavior, that is typically indicative of future behavior patterns, and with that data, we can create models to predict whether they will adhere to their care plan and can improve engagement in their treatment in a more personalized way.
For those that are highly engaged, the medication adherence tool that alerts them when they should dose may be a sufficient reminder. For others, their behavior may indicate that direct contact from the site is necessary for them to adhere to the protocol, while others may demonstrate that they will not be engaged regardless of outreach. This allows sites to tailor their outreach, rather than taking a blanket approach to all participants. Ultimately, this allows for better resource allocation and greater trial engagement.
OSP: Did you have anything to add?
RC: Having a deeper understanding of a patient’s lived experience with their treatment is increasingly important to a drug’s market success. By having consistent, accessible tools across both research and real-world deployment, we can tie back to all the predictive learnings from the research phase and apply them to personalize and predict a patient’s health journey.
As these algorithms continue to mature with more high-quality data, creating a predictive model that paints a picture of what it means to benefit from a medication opens the door to treating with unprecedented precision across the lifecycle of a drug.