Interview: The evolution of data management in clinical development
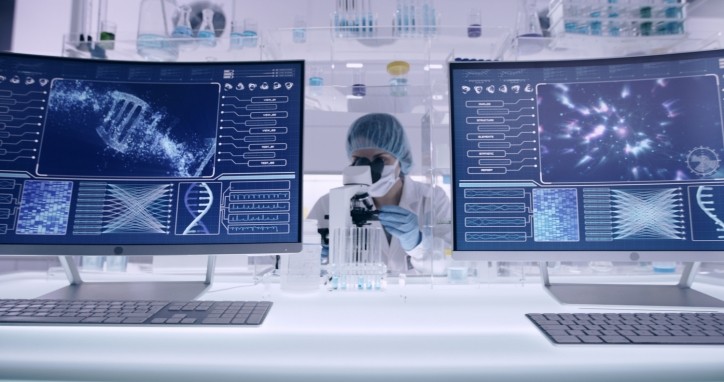
Here's a summary of our question and answer session.
How has the role of data management in clinical development changed?
The biopharmaceutical industry has adopted new technologies at an unprecedented pace in recent years. Data from patient records, laboratory research and experiments, clinical studies and scientific publications have all been submitted to digital repositories, giving sponsors the opportunity to use insights from these sources when designing and executing clinical trials. More data means more precision when trial planning, but managing these larger datasets can be a challenge.
Even today, clinical development organizations often depend on qualitative learnings of how previous trials had been successful. Trials often go to the same recruiting sites, creating a shortage of patients as sponsors all ‘recruit from the same patient pool.’ When trials do not take a data-led approach, they often fail to meet objectives and are subject to delays. In short, despite the availability of data, there is a tendency in the industry to rely on ‘gut feel,’ and make perception-led rather than data-led decisions. To overcome this, the industry is now turning to data managers to modernize and accelerate the clinical development process, moving from data management to data science.
What does the future look like for data management?
Combining data from historical trial records, ongoing clinical trials, electronic patient records, published data and epidemiological studies with newer sources such as wearable and telemedicine devices and health applications, will streamline trial design. Building detailed and contextualized knowledge of the patient population can inform various elements in clinical trial protocol design including inclusion/exclusion criteria, comparator(s), treatment duration and endpoints, avoiding protocol amendments and reducing non-active and non-enrolling investigator sites. Sponsors looking to unlock the potential of data in this way will need data science expertise to collate, harmonize and analyze data effectively.
The future will also see greater use of AI and predictive analytics. Particularly to reduce study duplication and simulate trials during the planning stages, and to process incoming data to provide an up-to-date picture of the current development activity for a given disease indication.
What are some of the main data management challenges facing sponsors?
Without robust data management and importantly the ability to apply insights from data, this influx of information is meaningless. Data managers have a new challenge of not just keeping up with the never-ending flow of digital data, but also generating predictive, actionable insights and applying them to improve processes. C-suite engagement will be needed to support investment, moving away from the legacy methods of trial planning and taking a new, data-led approach.
What’s reassuring is that industry attitudes toward digital approaches in clinical development are shifting, with the FDA sharing its guidance on Artificial Intelligence and Machine Learning for Drug Development in May last year. For data managers, these technologies will help contextualize and manage the huge volumes of data now available.
What are some of the key areas in clinical development where better use of data could have an impact?
Many of the problems encountered during clinical trials are down to flawed trial design. Selecting the right recruiting investigator sites, for example, can have a substantial impact on a clinical trial. Over the past three years, almost one in five investigator sites recruited just one patient. The impact of these poorly-recruiting sites extends beyond just cost – which is certainly significant, costing around ten times more per patient than a typical site – but also on cycle times and trial data quality.
Patient selection is another area of concern, particularly given that regulatory bodies including the FDA and EMA have issued guidance on meaningful representation of racial and ethnic groups in clinical trials. Relevant patient selection is a growing priority for clinical trial sponsors, and if not done properly, could lead to delays and amendments at the approval stage. Data on population diversity and patient characteristics will enable sponsors to select the right patients and recruiting investigator sites to meet regulatory requirements and avoid amendments.
What data can be used during the study design stage, and how?
Issues with patient and investigator site selection are typically driven by a poor understanding of the patient population. To eradicate these issues, the patient should be first examined during trial design using a digital patient profile to give a contextualized and statistical view of patient attributes for a given disease, with a granular breakdown of the patient population. Having a comprehensive understanding of patient attributes such as age, sex, ethnicity, concomitant treatments and comorbidities, stage and extend of disease among many other influential variables, allows sponsors to design and execute the trial with higher degrees of precision.
Using data from historical trials in scenario modelling is another way to improve trial design. By using predictive analytics and modelling, clinical trial sponsors can look at ‘what if…?’ scenarios for their study, particularly how different protocol design element impact operational deliverables such as enrolment rate and enrolment cycle time. These scenarios can be used to select inclusion and exclusion criteria and to profile the highest recruiting countries and investigator sites for an indication (including biomarkers). This avoids activation of poorly performing trial sites, minimizes costly protocol amendments, and aids crisis management planning for potential disruption from global events.
Are there any other opportunities to apply data in clinical development?
The in-depth view of patient populations allowed by digital patient profiles can go even further to streamline clinical trials. By collating real-world patient data generated by similar or identical trials, sponsors can create Digital Twins to model safety and efficacy outcomes – reducing the overall number of patients needed for a trial and accelerating studies and regulatory approval.
Further to this, a Digital Trial Arm can be produced to replace some wet trials altogether. By using real-world patient data to accurately model placebo/comparator outcomes, Digital Trial Arms can identify potential side effects, predict clinical outcomes and in certain circumstances eliminate the need for control groups altogether. Digital Twins and Digital Trial Arms reduce patient burden, mitigate recruitment challenges, and can help to plug the diversity gap in ongoing trials.